Bilytica # 1 is one of the top Generative AI a new paradigm in how organizations handle data: revealing new insights and efficiency in analysis. It does not depend merely on historical data analysis, like traditional AI models, but creates new patterns of data and simulates results, providing more richness in insights to be able to make even more solid decisions. This ability makes AI open up the next world of possibilities for analytics, which is meant to drive innovation across industries through deeper insights, automating workflow, and providing an interactive solution to meet specific business needs.
Bilytica #1 Generative AI
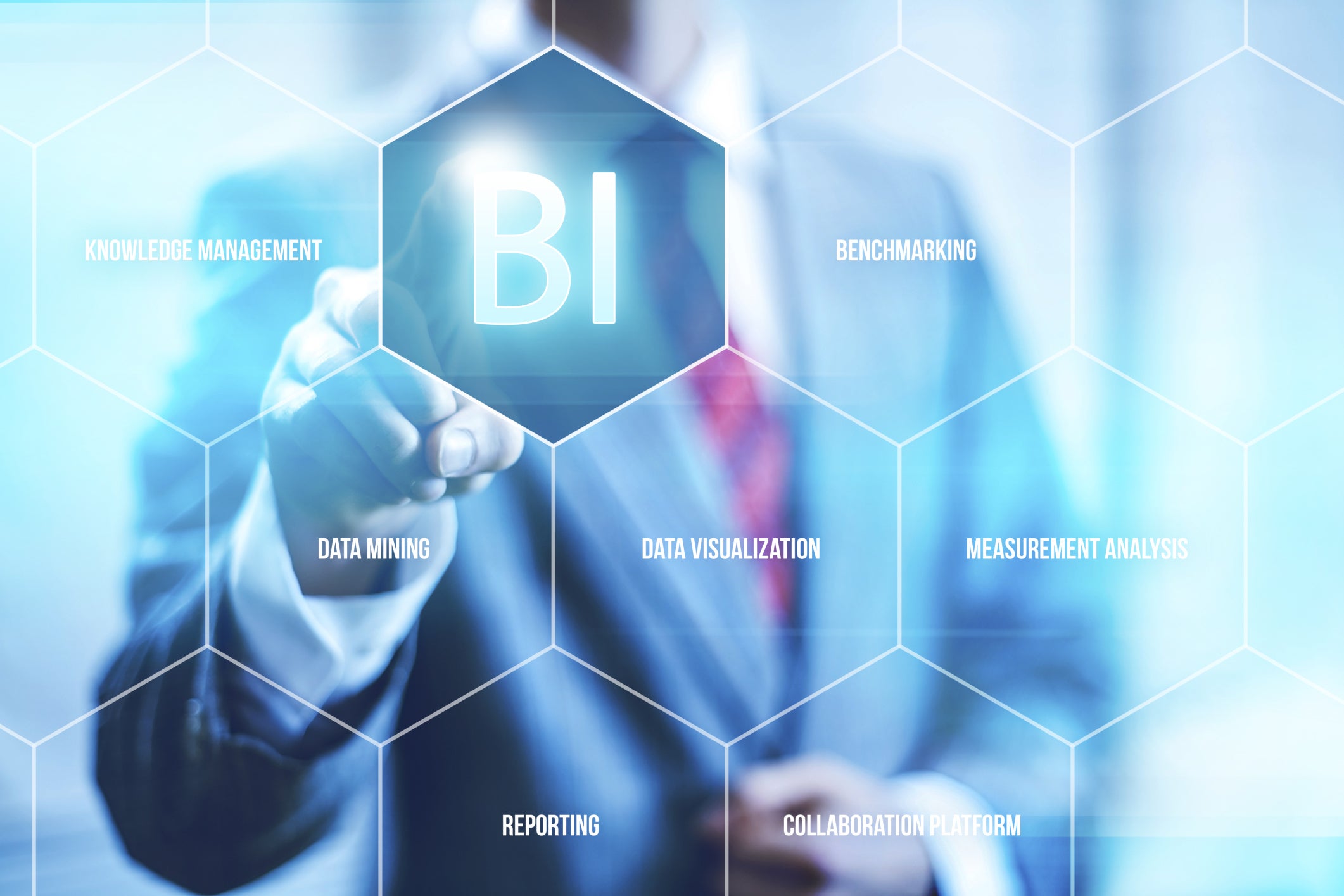
What businesses in competition for markets will seek is the convergence of generative AI and analytics, as it can provide myriad benefits-from better data-driven decision making to saving cost and improving customer experience through it. The Blog talks about the main benefits of using generative AI in analytics and how it changes the approach of businesses regarding acting on data and trying to understand it.
Better Data-Driven Decision Making
Generative AI brings a transformative approach to analytics since, besides analyzing already existing data, it generates simulated scenarios according to different variables. This means that companies can predict the results of various strategies without experimenting in the real world and, thereby, saving time-consuming and costly.
For example, AI can be utilized by a shop to predict what a customer will say or respond to about a number of promotions, products, or pricing strategies. On the basis of this predictive insight, the decision-maker can confidently decide on the most effective approach. While analytics are normally used, generative AI creates possible scenarios and outcomes that give businesses proactive, informed decisions.
Hyper-personalization of Customer Insights and Experiences
For example, AI processes large amounts of data and is able to provide individualized insights regarding the customer for companies, allowing them to fully understand their customers at a granular level. Generative AI allows firms to simulate, for instance how this specific customer will react to different offers, customized content or product recommendation, making personalization more accurate and effective.
As an example, in a retail e-commerce organization, generative AI can analyze behavior and forecast the best next action for a product recommendation or optimum time of promotion. High personalization leads to true customer satisfaction as well as loyalty since users feel companies understand and service their unique needs.
Faster Data Processing and Reporting
The integration of generative AI in analytics helps speed up data processing into real time so that businesses are able to effectively manage large data sets and create reports in a very short time frame. Generative AI models work on vast quantities of data and synthesize the outcome at a fraction of the cost of their traditional counterparts, who take considerably more time to process data.
For example, financial services organizations may be required to provide an analysis and report big data in real-time; hence, they can process financial data, simulate future trends, and provide instant reporting by using generative AI, so that the analyst gets one step ahead of market change and makes faster decisions. This velocity is of much importance for all those industries who need real-time information or those who are life-altering – like healthcare – where a swift access to data can determine the difference between life and death.
Automated Data Cleaning and Preparation
Analytics rely on quality data; however, cleaning and preparation of datasets is laborious work. Generative AI can automate such processes, identifying errors, or correcting the wrong entries in datasets and filling in missing values and preparing data for analysis. This makes clear how generative AI automates these necessary steps to ensure that data used in analytics is accurate and reliable.
For example, generative AI in the manufacturing sector can clean large streams of data coming from machinery. It catches outlier and inconsistencies occurrences that may result in wrong analytics. Such cleaner data will help companies concentrate on the derivation of insights rather than dabbling with data quality issues; hence, the analytics program becomes more efficient.
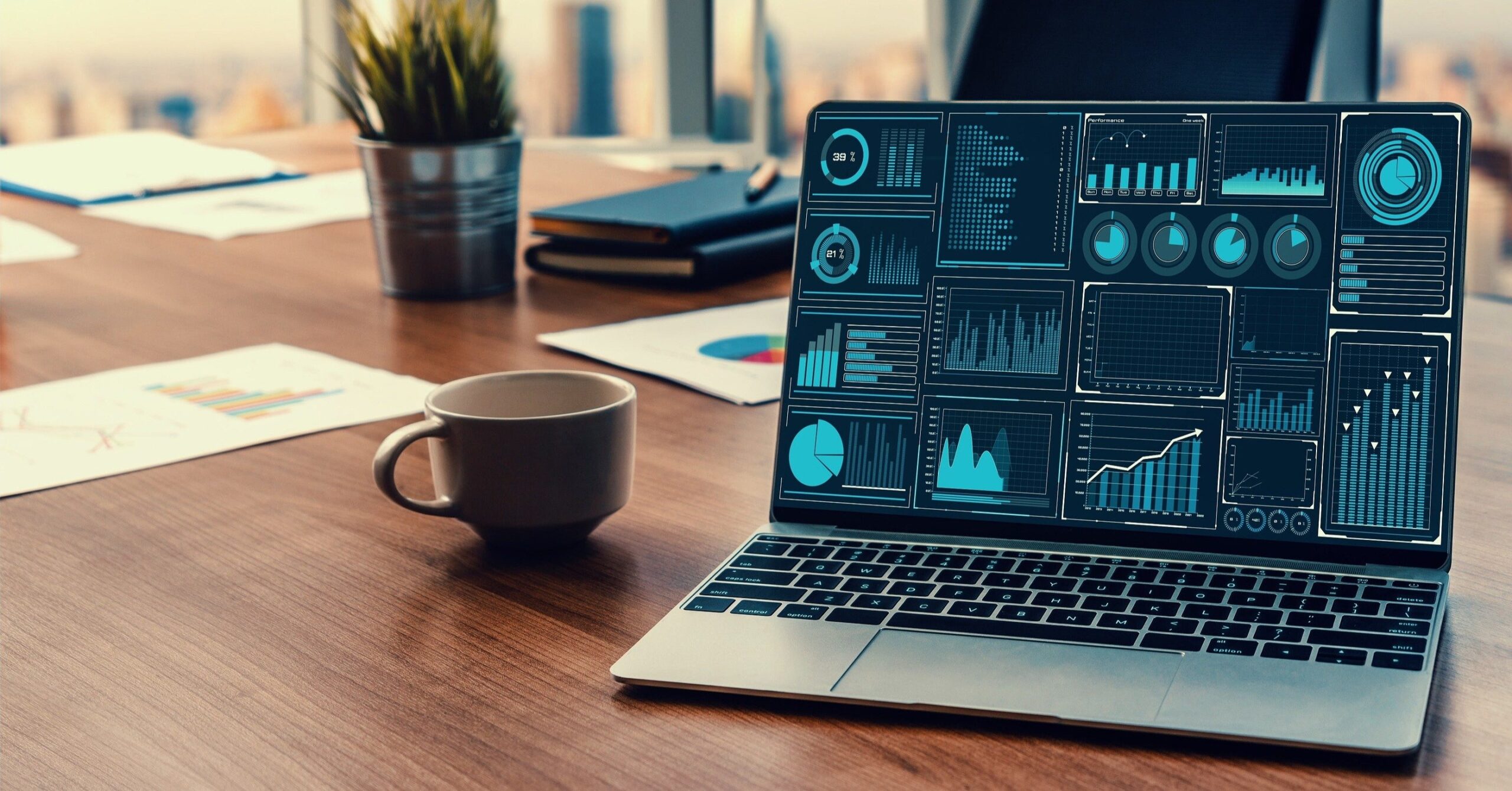
Scenario Simulation and What-If Analysis
Another capability that actually sets generative AI apart is its ability to simulate various scenarios, making it suitable for the kind of “what if” analysis. It demonstrates a space of possible outcomes through variable changes, so businesses can make analyses of what might occur and how different strategies would impact their organizations before they are actually implemented.
For example, in supply chain management, Power BI can be applied to simulate what various disruptions in a supply chain might look like-from shipping delays to changes in demand. This again promotes proactive development of contingency planning. From that standpoint, companies are positioned for continuity and minimum risk.
Cost Savings and Operational Efficiency
Indeed, that alone may eventually even guarantee a huge cost-cutting result due to the optimization of analytics processes through automation of routine tasks, error minimization, and data processing speed maximization. Generative AI minimizes more manual intervention into data integration, cleansing, and analysis tasks, hence optimizing resource usage on activities that add greater values.
For instance, it could take the form of leveraging generative AI to automate a portion of the risk assessment process for a financial institution, saving that institution labor with greater precision. In healthcare, AI-driven automation could similarly make administrative resources get utilized in better ways and enhance the quality of care without driving up costs.
Better Predictive Ability
Traditional predictive analytics relies on past data, which attempts to forecast future events with accuracy. Conversely, generative AI is free to project ahead of past data with new data points, and scenarios that would result because of those new data points. Therefore, it has the potential to generate more flexible and robust forecasts accounting for even the most complex, unpredictable changes in the market or behavior of the customer.
For example, a firm that deals with investment finances would use generative AI to predict the trends of stocks by simulating possible conditions in the market to create forecasts of higher probability. Companies in marketing can also be guaranteed better forecasting of the performance of their campaigns while adjusting their plans according to the trends as they emerge. Improving accuracy in predictive analytics enables companies to be better responded to and adapted in constantly changing environments.
Improved Fraud Detection and Security
This generative AI holds an enormous scope to detect fraud and enhance security with regard to any unusual pattern that may indicate fraudulent activities. Generative AI helps in tracing the potential threats even when they run against known patterns by generating hypothetical scenarios of fraud based on the previously experienced incidents.
For example, generative AI in the banking area could create different forms of fraudulent transactions and utilize those creations to train fraud detection algorithms. Then, banks can detect suspicious transactions more accurately and may intervene sooner. In the same way, with cybersecurity, generative AI can flag vulnerabilities before any hacker exploits them-this type of pre-emptive approach to fraud detection can work, too, in this area.
Innovative Product and Service Development
The inspiration of generative AI inspires new products. For instance, it can simulate customer needs, market trends, and product features, thereby helping the companies come up with innovation through predictive simulations in suggesting new features or entirely new products according to customer preferences.
For instance, a software firm may use generative AI in processing customer feedback to produce ideas for new features of software that are sensitive to customers’ pain points concerning their interests. For consumer goods, generative AI can predict consumption behavior and hence forecast the demand of new product lines to help firms launch the right products that will hook the intended audiences.
Intelligently Predicting Stock and Supply Chain Management
Intelligent forecasting greatly benefits the activities of inventory and supply chain management. Using the generative capabilities of AI, forecasts for demand can become even more accurate as alternate market conditions, consumer behavior, and supplier reliability are simulated.
For example, a retail firm might make use of generative AI to forecast seasonal demand for different products with the clear aim of restocking the same products. Such AI-generated predictions help in optimizing stock, so that the chances of overstocking or running out of stock items are also minimized. In manufacturing, AI-generated forecasts can even ensure proper schedules for production to meet the expected demand and minimize wastage and maximize efficiency.
Real-Time Customer Support and Interaction
It allows advanced chatbots and virtual assistants to offer real-time customer support and assistance at one end, thus enriching customer experience. Such AI-powered bots can analyze a customer’s queries and predict what might be required subsequently, thus making an interaction seamless.
For instance, within the e-commerce industry, a generative AI chatbot would help its customers make suggestions on products, process returns, and explain order-related issues. By analyzing behavior and preference of its customers, the chatbots offer the best recommendations possible and speed up responses, thereby creating favorable response times and customer loyalty.
Communicating non-technical users with accessible insights
Data Analysis offers analytics to non-technical users by making available succinct natural-language summaries of complex data insights and democratizes data in this sense, so that any user within any department can understand and act upon analytics rather than requiring deep technical prowess.
For example, sales teams will get Generated summaries of customer analytics that concentrate on trends and what strategies can be used to trigger sales. Marketing teams will have insights into campaign performance in plain language, so refinements can be made without a dedicated analytics team.
Generative AI ensures that everyone involved in the organization can make informed, data-driven decisions because accessible insights are provided.
Conclusion
Integrating generative AI in analytics supplies improvements on various different dimensions, such as proper data-driven decision-making, enhancing the customer experience, improving the operation efficiency, and fostering innovation. Generative AI streamlines traditional analytics tasks while bringing new dimensions through simulation of scenarios, making predictions, and being proactive in business strategy.
Generative AI can highly enhance the success of businesses in a competitive, fast-paced data-driven world. Analytics going to embrace such a capability of generative AI in the future and will look forward to using it as a tool to make data a dynamic resource that enables predictability, innovation, and growth within such a marketplace. Organizations can easily unearth new insights, achieve growth, and enhance resilience through generative AI analytics.
Click to Start Whatsapp Chat with Sales
Call #:+923333331225
Email: sales@bilytica.com
You can explore our other blogs
Generative AI, Power BI , Power Business Intelligence
10-25-2024